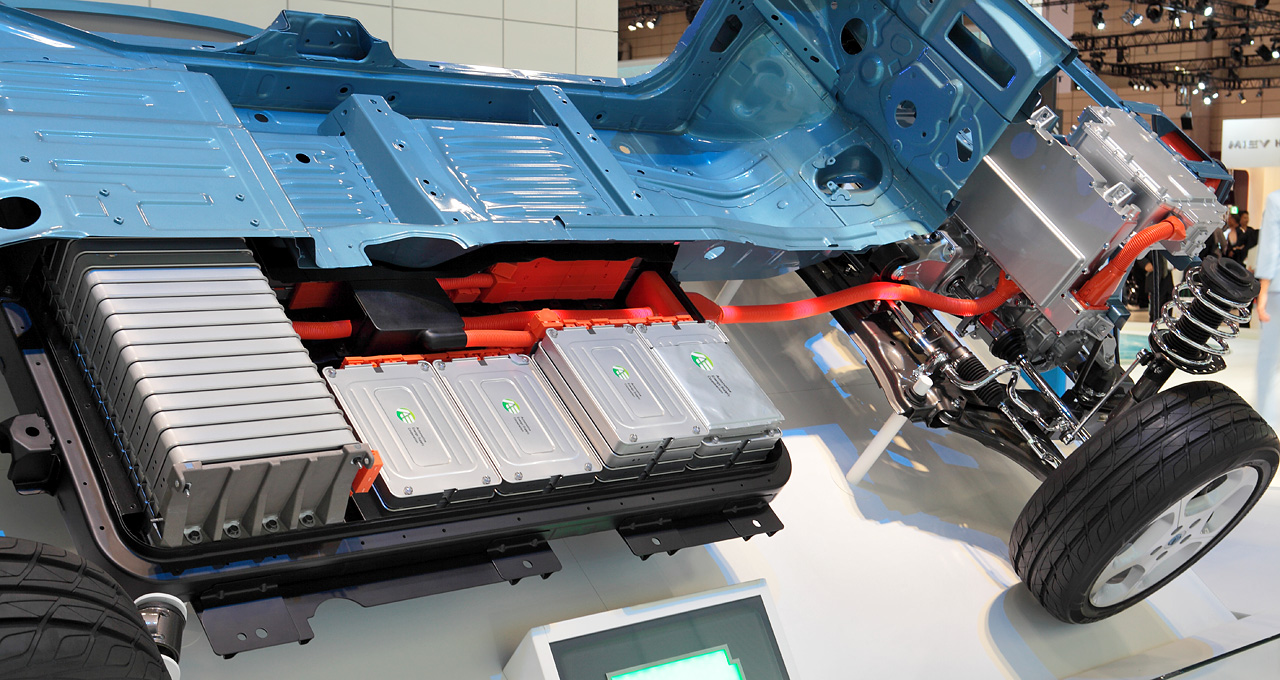
Renewable energies, such as wind and solar energy are naturally intermittent. To balance their demand and supply, batteries of, for example, electric vehicles can be charged and act as an energy buffer for the power grid. Cars spend most of their time idle and could, at the same time, feed their electricity back into the grid. While this is still a dream of the future, commercialization of electric and hybrid vehicles is already creating a growing demand for long-lasting batteries, both for driving as well as grid buffering. Consequently, methods for evaluating the state of the battery will become increasingly important.
The long duration of battery health tests is a problem, hindering the rapid development of new batteries. Better battery life forcasting methods are therefore urgently needed but are extremely difficult to develop. Now, Severson and her colleagues report in the journal Nature Energy that machine learning can help to predict computer battery life by creating computer models. The published algorithms use data from early-stage charge and discharge cycles.
Normally, a figure of merit describes the health of a battery. It quantifies the ability of the battery to store energy relative to its original state. The health status is 100% when the battery is new and decreases with time. This is similar to the state of charge of a battery. Estimating the state of charge of a battery is, in turn, important to ensure safe and correct use. However, there is no consensus in the industry and science as to what exactly a battery’s health status is or how it should be determined.
The state of health of a battery reflects two signs of aging: progressive capacity decline and impedance increase (another measure of electrical resistance). Estimates of the state of charge of a battery must therefore take into account both the drop in capacity and the increase in impedance.
Lithium ion batteries, however, are complex systems in which both capacity fade and impedance increase are caused by multiple interacting processes. Most of these processes cannot be studied independently since they often occur in simultaneously. The state of health can therefore not be determined from a single direct measurement. Conventional health assessment methods include examining the interactions between the electrodes of a battery. Since such methods often intervene directly in the system “battery”, they make the battery useless, which is hardly desired.
A battery’s health status can also be determined in less invasive ways, for example using adaptive models and experimental techniques. Adaptive models learn from recorded battery performance data and adjust themselves. They are useful if system-specific battery information are not available. Such models are suitable for the diagnosis of aging processes. The main problem, however, is that they must be trained with experimental data before they can be used to determine the current capacity of a battery.
Experimental techniques are used to evaluate certain physical processes and failure mechanisms. This allows the rate of future capacity loss to be estimated. Unfortunately, these methods can not detect any intermittent errors. Alternative techniques use the rate of voltage or capacitance change (rather than raw voltage and current data). In order to accelerate the development of battery technology, further methods need to be found which can accurately predict the life of the batteries.
Severson and her colleagues have created a comprehensive data set that includes the performance data of 124 commercial lithium-ion batteries during their charge and discharge cycles. The authors used a variety of rapid charging conditions with identical discharge conditions. This method caused a change of the battery lives. The data covered a wide range of 150 to 2,300 cycles.
The researchers then used machine learning algorithms to analyze the data, creating models that can reliably predict battery life. After the first 100 cycles of each experimentally characterized battery their model already showed clear signs of a capacity fade. The best model could predict the lifetime of about 91% data sets studied in the study. Using the first five cycles, batteries could be classified into categories with short (<550 cycles) or long lifetimes.
The researchers’ work shows that data-driven modeling using machine learning allows forecasting the state of health of lithium-ion batteries. The models can identify aging processes that do not otherwise apparent in capacity data during early cycles. Accordingly, the new approach complements the previous predictive models. But at Frontis Energy, we also see the ability to combine generated data with models that predict the behavior of other complex dynamic systems.
(Photo: Wikipedia)